Amazing Info About How To Determine Line Of Best Fit Pyplot Chart
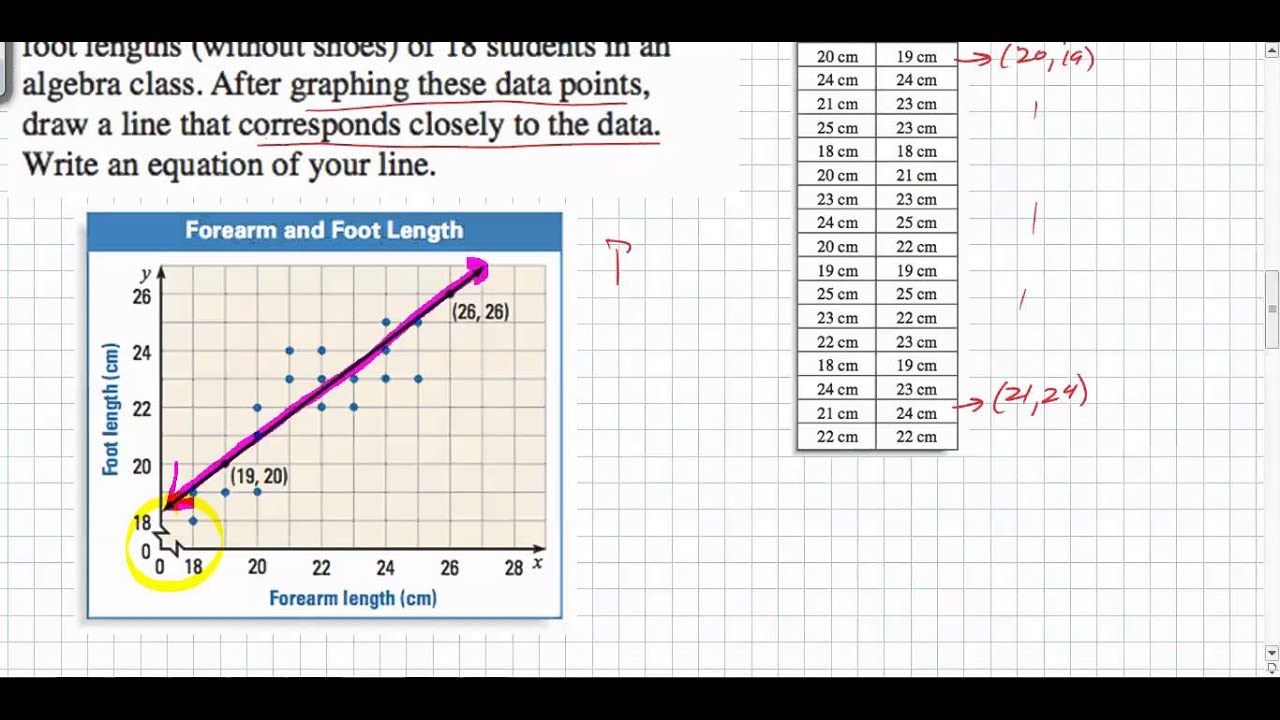
Learn how to approximate the line of best fit and find the equation of the line.
How to determine line of best fit. (b) use your answer to part (a) to estimate the value of q when p = 4. We go through an example in this free math video tutorial by mario's. Eyeball method, point slope formula, or least square method.
Then drag the red line to find the line of best fit. Y = c + b1(x1) + b2(x2) where, y is dependent variable. A line was fit to the data to model the relationship.
Understanding positive correlation and negative correlation. (a) find the equation of the line of best fit in terms of q and p. Don’t just choose the first and last data points, but construct a line that best represents the trend.
That is, we need to find the values b 0 and b 1 that minimize: For example, dots at (3,5),(6,6),(7,8) can have a line run through their main path that they look like they head towards. Not all lines of best fit hit all the points.
Beginning with straight lines; (c) estimate the value of p when q = 3. A line of best fit is a straight line that minimizes the distance between it and some data.
I want to be able to do this manually, not with graphing tools. Use a graphing utility to find the line of best fit. A line of best fit is used to show a trend between points.
First, we must construct a scatter plot from the given data and understand correlation. Q = ∑ i = 1 n ( y i − y ^ i) 2. The term “best fit” means that the line is as close to all points (with each point representing both variables for a single person) in the scatterplot as possible, with a balance of scores above and below the line.
A line of best fit is a straight line that shows the relationship between two sets of data. Line of best fit in linear regression. Fitting a line by eye residuals;
We can use the line to make predictions. The line of best fit is used to express a relationship in a scatter plot of different data points. Explore math with our beautiful, free online graphing calculator.
It helps us predict results based on an existing set of data as well as clear anomalies in our data. Give the equation in its simplest form. Y ^ i = b 0 + b 1 x i.